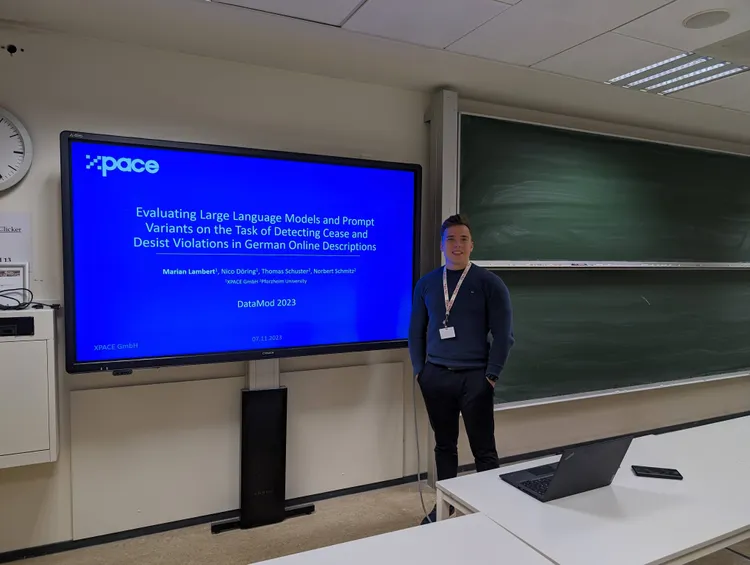
Evaluating Large Language Models and Prompt Variants on the Task of Detecting Cease and Desist Violations in German Online Product Descriptions
DataMod 2023
In my talk at DataMod 2023, I discussed our paper titled “Evaluating Large Language Models and Prompt Variants on the Task of Detecting Cease and Desist Violations in German Online Product Descriptions.” This research, conducted as part of the KIVEDU project, focused on comparing various large language models (LLMs) in identifying violations of cease and desist declarations in German online product descriptions.
We evaluated two proprietary models by OpenAI (gpt-3.5-turbo-0301 and gpt-3.5-turbo-0613) and three open source models (LLaMA2, StableBeluga2, and Platypus2). Our methodology involved using different prompt variations on a dataset of 116 manually labeled pairs of cease and desist declarations and product descriptions.
Our research aimed to answer two key questions: Which LLM is most adept at identifying violations, and how do prompt variations impact model performance? We discovered that StableBeluga2 was the most effective model, achieving the highest accuracy and micro F1 score. It was also the most reliable, showing minimal deviations in performance across different prompt variants. Platypus2 and gpt-3.5-turbo-0301 also performed well, though their performance varied more significantly. LLaMA2 was the least effective.
We found that prompt variations significantly influenced model performance. The presence of step-by-step instructions generally decreased performance, while a “yes”/“no” output format led to higher performance. However, this was highly model-specific. Role prompting and providing longer versus shorter instructions had minimal impact on performance across all models.
Overall, the study demonstrated the potential of LLMs in automating the detection of cease and desist violations in online product descriptions, although further research is needed to evaluate other models and prompt variations, as well as to explore approaches like LLM fine-tuning on domain-specific data for improved performance.
I thoroughly enjoyed giving this talk and meeting everyone at the event. The interactions and insights shared by the attendees were invaluable, and it was a fantastic opportunity to engage with others who are passionate about the intersection of AI, language models, and legal applications.